Machine learning and artificial intelligence has permeated much of everyday life. From ChatGPT, which can write college essays and cover letters, to ZeroEyes, an AI-led company that monitors for potential mass shootings, the technology is being used for both small- and large-scale use.
It’s not surprising, then, that lenders have used machine learning predictions to influence lending decisions for mortgages, personal loans and the like. Advances in AI technology have helped lenders predict default for applicants, but they haven’t really made access to credit more equitable, the Federal Reserve Bank of Philadelphia found in recent research.
A working paper from the Philly Fed, titled “One Threshold Doesn’t Fit All: Tailoring Machine Learning Predictions of Consumer Default for Lower-Income Areas,” explains this research and makes a suggestion toward achieving more equity in the process. Authors Vitaly Meursault, Daniel Moulton, Larry Santucci and Nathan Schor, published the work in November; Technical.ly spoke with Meursault last week to understand its local relevance.
The research looks at people in low- and moderate-income areas. In Philadelphia, that accounts for 45% of the city, or about 700,000 people. The researchers used fairness machine learning literature to inform a suggestion to making AI lending more equitable: Reducing credit score thresholds in these low- and moderate-income neighborhoods.
Research published by The Journal of Finance and cited in the working paper showed that people of color overall faced more uncertainty from lenders assessing their credit. It also showed that Black and Latinx borrowers benefited less with sophisticated learning models assessing lending options. Underserved and underbanked populations tended to have lower credit scores, and these low- and moderate-income neighborhoods saw worse outcomes by predictive technology.
“For lending decisions based solely on credit scores, this means that in [low- and moderate-income] areas consumers who should receive credit are relatively less likely to get it, while other consumers end up with loans they might not be able to pay back,” the paper said.
Read the working paperFor Meursault, a machine learning economist for the Philly Fed, there’s no question of whether lending companies are using AI and machine learning tools.
“We believe it’s a great time to be thinking not just about if they’re going to use these models — because they are — but also how we can guide the use of these models,” he said.
Those who work to remove bias from machine learning do so in about three ways: They can find ways to correct bias within a data set, they can work to train the modeling to work in specific ways, or they can apply fairness constraints in modeling, essentially changing how you’ll use the prediction. Implementing the use of a lower credit score requirement in low- and moderate-income neighborhoods is an example of this third practice.
“We have to reconcile the interests of different stakeholders,” Meursault said. “Regulators want lending to be more fair and lenders want higher profits.”
The paper is meant to be a conversation starter, the economist said, especially with academics, industry practitioners and regulators. It argues that interest in fair credit, lending and technology goes back to the 1970s, with the passing of the Equal Credit Opportunity Act of 1974. Meursault said that the team is trying to show that simple techniques for guiding machine learning models exist and aren’t hard to implement from a technical standpoint.
“With advances in AI, we have choices,” he said. “To ban it, to accept them as they are, or to guide their use to achieve outcomes that are more consistent with our values.”
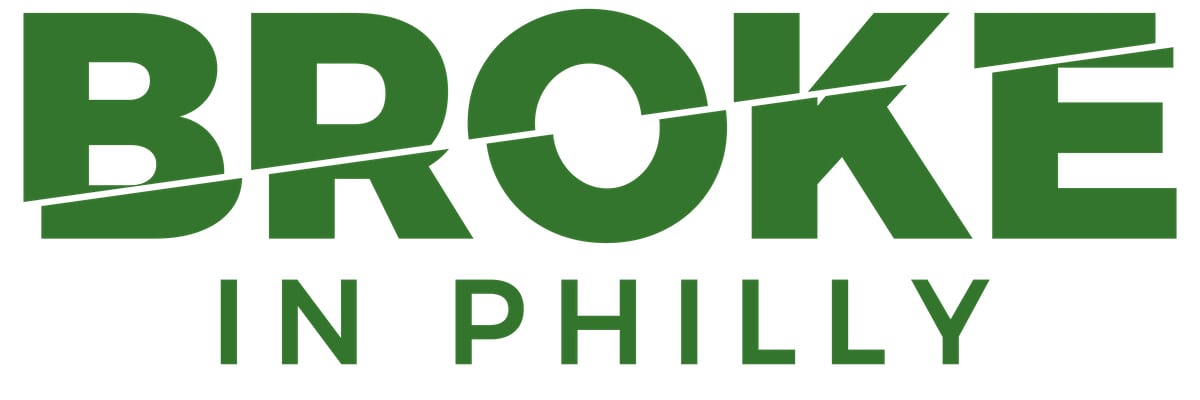
Technical.ly is one of 20+ news organizations producing Broke in Philly, a collaborative reporting project on solutions to poverty and the city’s push toward economic justice.
Before you go...
Please consider supporting Technical.ly to keep our independent journalism strong. Unlike most business-focused media outlets, we don’t have a paywall. Instead, we count on your personal and organizational support.
Join our growing Slack community
Join 5,000 tech professionals and entrepreneurs in our community Slack today!

The person charged in the UnitedHealthcare CEO shooting had a ton of tech connections

From rejection to innovation: How I built a tool to beat AI hiring algorithms at their own game

How a laid-off AI enthusiast pivoted to become a founder — while holding down a day job
