To be sure, as we learned from more than a dozen technologists gathered by Technical.ly for a moderated Zoom conversation in tandem with Spotify, there’s quite a lot to like and dislike about this ever-changing technological frontier. The ways it impacts our work range from the obvious to the subtle, from the attention-grabbing to the mundane, across all ethical categories and considerations.
Here’s just a sample of what these technologists shared during the conversation.
Ethical quandaries
First, the bad. The effects of machine learning and the processes associated with it (i.e. artificial intelligence, optimization, etc.) on our lives are a constant subject of critique, especially given the various moral issues they conjure. Maurice Gaston, a software development manager at Philly HQ’d Comcast, captured those issues in a succinct reflection.
“We’re all being trained by the ML that are helping us out,” he said. “We are being trained how to use these systems rather than having the systems learn how we work.”
Other participants spoke to the murky line between when a machine learning system’s biases might reflect our own, and how to deal with that. Kevin Kho, a Chicago-based technologist, said that he used to work for a payroll company that used machine learning to help clients predict which of their employees may leave based on pay. He and his colleagues had to work with the employers’ ethics team to determine which variables, especially demographic ones like race or gender, could be used.
“We were intentionally not allowed by our internal ethics committee to use these kinds of variables,” he noted.
That ethical picture becomes even cloudier when considering how far behind regulators are compared to the companies whose tech they’re supposed to moderate. Katya Willard, a senior machine learning engineer at Spotify, touched on the ambiguities that have made such policies as the European Union’s General Data Protection Regulation so confusing to navigate.
“It’s written by people who don’t really know how things [technologically] work, and now we’re all dealing with the fallout of it and trying to parse out what to do,” she said. “It’s really hard.”
The ubiquity
One point that repeatedly arose is that, despite these controversies, machine learning is embedded in how we operate online, even if it’s not a core part of people’s work.
For instance, Salas Saraiya — the cofounder and CTO of Philadelphia-based Employee Cycle, as well as one of several 2022 RealLIST Engineers who took part in the conversation — noted how much machine learning matters to social media algorithms.
“I go on Twitter, and whatever it decides to surface, it’s all ML,” he said. Eliot Pearson, the CTO of Baltimore’s Enradius, discussed how he used predictive analytics while working for Yahoo nearly a decade ago, at a time when ML tech was not as mainstream. There, the analytics aimed to help customers “get the things they want to see all the time” in front of them as much as possible, he said.
“It allowed us to keep our customers and make them happy, so it made our jobs easier as producers of solutions for people,” he said.
Behind the scenes, machine learning processes aid technologists in ways that front-end users may never see. UX designer and PhillyCHI board member Robin Zheng spoke about how she’s trying to help her employer, the data professionals training entity Correlation One, better match its program participants with career coaches using a matching algorithm.
“Right now, it’s a super manual process, [maintained] manually across several spreadsheets by our program managers,” she said. “This helps us run the service better, but the actual end user, like our fellows or students, wouldn’t actually see this.
Baltimore-based Barry Wright, a group product manager for Spotify, added that this kind of backend work is where machine learning matters, even if it doesn’t generate the most hype.
“People see the news headlines about AI-generated art or ML projects, but if you got into it, the iceberg is all little internal things that make life and work in a company easier or better,” he said. “Cleaning up of data or optimization processes — it’s not as exciting to write an article about, but it’s where a lot of this work is actually getting done. That’s the starting point for a lot of the interesting work that you see later on.
Join the conversation!
Find news, events, jobs and people who share your interests on Technical.ly's open community Slack

Delaware daily roundup: Vital conditions framework; Seaford center breaks ground; Staff shortage at the beach

Delaware daily roundup: Equity Action Summit video; DE Senate debates wind energy; Hydrogen hub listening sessions

Philly daily roundup: Student-made college cost app; Central High is robotics world champ; Internet subsidy expiration looms
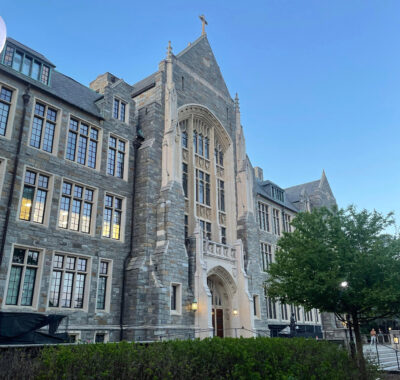